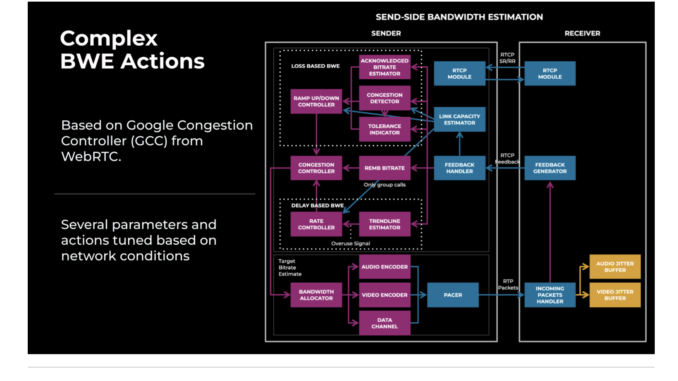
[ad_1]
Researchers from Meta developed a machine learning (ML)-based approach to address the challenges of optimizing bandwidth estimation (BWE) and congestion control for real-time communication (RTC) across Meta’s family of apps. Existing techniques, such as WebRTC’s Google Congestion Controller (GCC), rely on hand-tuned parameters, leading to complexities and inefficiencies in handling diverse network conditions. Maintaining a trade-off between quality and reliability. When one aspect is increased, the other is compromised, making optimizing the user experience across different network types difficult.
The existing BWE module at Meta is based on GCC, which uses parameter tuning to enhance performance. However, this has resulted in a complex system with multiple parameters and actions dependent on network conditions. Meta’s proposed method targets network problems holistically across various layers, including BWE, network resiliency, and transport. The approach aims to replace hand-tuned rules with a simpler alternative, leveraging time series data for offline parameter tuning and network characterization.
Meta’s ML-based approach involves two main components: offline ML model learning and parameter tuning. The model learning phase utilizes time series data from production calls and simulations to categorize network types and optimize parameters. The architecture combines LSTM layers for processing time series data and dense layers for non-time series data, enabling accurate modeling of network conditions. For instance, in the case of random packet loss classification, the ML model detects such losses and adjusts BWE parameters accordingly to increase tolerance and improve network resiliency. Furthermore, the ML model predicts congestion in low-bandwidth scenarios, allowing proactive optimization to prevent video freezes and connection drops.
The experiment results demonstrate significant improvements in reliability and quality metrics across different network types, highlighting the effectiveness of the ML-based approach. For instance, congestion prediction reduces connection drop rates and enhances user experience, while BWE optimization improves video quality and reduces freeze percentages. The result demonstrates the superiority of ML solutions over traditional hand-tuned rules for networking, especially in targeting, monitoring, and updating network conditions efficiently.
In conclusion, Meta’s new approach presents a novel ML-based approach to address the challenges of BWE and congestion control in RTC applications. By leveraging time series data and offline parameter tuning, the proposed method achieves significant improvements in reliability, quality, and user engagement metrics across diverse network conditions. The performance of ML solutions heavily depends on data quality and labeling, showcasing the importance of accurate training data for better results.
Pragati Jhunjhunwala is a consulting intern at MarktechPost. She is currently pursuing her B.Tech from the Indian Institute of Technology(IIT), Kharagpur. She is a tech enthusiast and has a keen interest in the scope of software and data science applications. She is always reading about the developments in different field of AI and ML.
[ad_2]
Source link
Be the first to comment